Introduction
Technology has revolutionized our lives, and the healthcare industry is no exception. Artificial Intelligence (AI) and Machine Learning (ML) are reshaping medical devices, enhancing patient care, and improving outcomes. In this B2B article, we explore the evolution of AI and ML in medical devices, considering global trends and the Indian market.
Artificial Intelligence (AI) and Machine Learning (ML) have revolutionized numerous industries, and healthcare is no exception. Medical devices enhanced by AI and ML are transforming diagnostics, treatment, and patient care. This article delves into the evolution of AI and ML in medical devices, focusing on recent research, market analysis, and the impact on global and Indian markets.
The Rise of AI and ML
Data-Driven Insights:
o AI/ML-enabled devices analyze vast data volumes, providing insights that were previously unattainable.
o From diagnostics to treatment planning, these technologies enhance decision-making.
Applications in Healthcare:
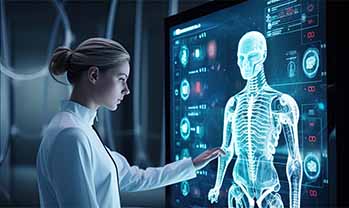
o AI/ML aids in disease detection (e.g., cancer, diabetes), personalized medicine, and drug development.
o Predictive analytics optimize hospital operations and patient management.
Regulatory Landscape
FDA and Beyond:
o The US FDA leads in defining regulatory frameworks for AI/ML medical devices.
o Global guidelines (e.g., WHO) influence national regulations.
Challenges:
o Transparency: AI algorithms differ from traditional technologies, demanding transparency.
o Data Quality: Bias can arise from incomplete or skewed datasets.
o Change Management: AI’s adaptive nature requires robust version control.
Market Trends
Global Market Growth:
o The AI in medical devices market reached $8.4 billion in 2023, growing at 21.99% CAGR since 2018.
o By 2028, it’s projected to reach $27.7 billion, with continued growth to $76.39 billion by 20331.
Indian Context:
o India’s healthcare sector embraces AI/ML for telemedicine, diagnostics, and rural healthcare.
o Startups and established players collaborate to drive innovation.
Diagnostic Tools
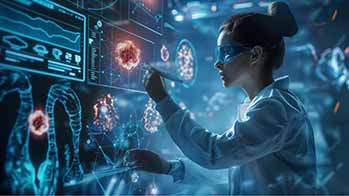
AI-powered diagnostic tools have become increasingly prevalent. These tools use ML algorithms to analyze medical images, detect anomalies, and assist in early diagnosis. For instance, AI algorithms can identify patterns in radiology images that are often missed by the human eye, significantly improving diagnostic accuracy.
Predictive Analytics
Predictive analytics in medical devices help anticipate patient outcomes based on historical data. This application is particularly useful in managing chronic diseases and predicting potential complications, allowing for proactive intervention.
Personalized Medicine
AI and ML are at the forefront of personalized medicine, offering tailored treatment plans based on individual patient data. These technologies analyze genetic information, lifestyle factors, and other data points to optimize treatment strategies, enhancing patient outcomes.
Market Analysis: Global Perspective
Growth Trends
The global AI in the healthcare market is experiencing exponential growth. According to recent reports, the market size is expected to reach $45.2 billion by 2026, growing at a compound annual growth rate (CAGR) of 44.9% from 2021 to 2026. This growth is driven by the increasing adoption of AI and ML technologies in various healthcare applications.
Key Players
Major players in the global market include IBM Watson Health, Google Health, and GE Healthcare. These companies are investing heavily in research and development to advance AI and ML capabilities in medical devices.
Regulatory Landscape
The regulatory environment is evolving to keep pace with technological advancements. Regulatory bodies like the FDA and the European Medicines Agency are developing frameworks to ensure the safety and efficacy of AI-powered medical devices.
Market Analysis: Indian Perspective
Adoption and Innovation
India is emerging as a significant player in the AI and ML medical devices market. With a growing healthcare sector and a large pool of skilled IT professionals, India is well-positioned to innovate and adopt these technologies. The Indian AI in healthcare market is projected to grow at a CAGR of 40% from 2021 to 2026.
Government Initiatives
The Indian government is actively promoting AI and ML in healthcare through various initiatives. Programs like the National Digital Health Mission (NDHM) aim to digitize healthcare records, providing a robust infrastructure for AI and ML applications.
Challenges and Opportunities
Despite the promising growth, challenges such as data privacy concerns, lack of standardization, and limited infrastructure in rural areas remain. However, these challenges also present opportunities for startups and established companies to develop innovative solutions tailored to the Indian market.
Recent Research and Developments
Breakthrough Studies
Recent studies have demonstrated the potential of AI and ML in improving patient outcomes. For example, a study published in Nature Medicine highlighted the use of AI algorithms to predict cardiovascular diseases with greater accuracy than traditional methods. Another study in The Lancet Digital Health showcased AI’s ability to diagnose eye diseases from retinal scans with high precision.
Collaborative Efforts
Collaborations between technology companies and healthcare institutions are driving innovation. For instance, Google’s partnership with Mayo Clinic aims to develop AI models for personalized cancer treatment. Such collaborations are crucial for translating research into practical applications.
The Future of AI and ML in Medical Devices
Emerging Technologies
Emerging technologies like quantum computing and advanced neural networks hold the potential to further enhance AI and ML capabilities in medical devices. These advancements could lead to more accurate diagnostics, efficient treatment plans, and improved patient care.
Ethical and Regulatory Considerations
As AI and ML technologies continue to evolve, ethical and regulatory considerations will become increasingly important. Ensuring patient data privacy, addressing algorithmic biases, and maintaining transparency in AI-driven decisions are critical for the sustainable growth of these technologies in healthcare.
Key Considerations
Ethical Implications:
o Balancing innovation with patient safety and privacy is crucial.
o AI/ML must align with ethical guidelines and patient consent.
Collaboration and Education:
o Industry collaboration fosters knowledge sharing and accelerates adoption.
o Continuous education for healthcare professionals ensures effective utilization.
Can you provide examples of successful AI/ML medical devices?
Imaging System for Skin Cancer Diagnosis:
o An imaging system that uses AI algorithms to provide diagnostic information for skin cancer in patients1.
Smart Sensor for Heart Attack Risk Estimation:
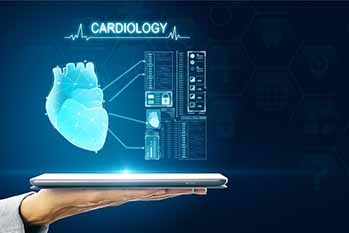
o A smart sensor device that estimates the probability of a heart attack based on physiological data1.
These devices demonstrate how AI and ML are transforming healthcare by enhancing diagnostics, risk assessment, and patient outcomes.
How accurate are these devices compared to traditional methods?
The accuracy of AI/ML medical devices varies depending on the specific application and the quality of data used for training. However, here are some general observations:
Imaging Diagnostics:
o AI algorithms can analyze medical images (e.g., X-rays, MRIs) with high accuracy.
o In some cases, AI outperforms human radiologists in detecting abnormalities.
Risk Prediction:
o Devices predicting heart attack risk or other health events achieve good accuracy.
o They consider multiple factors (e.g., vital signs, medical history) to provide personalized assessments.
Challenges:
o Data quality matters: Garbage in, garbage out. Reliable training data is essential.
o Interpretability: AI models can be “black boxes,” making it hard to explain their decisions.
In summary, AI/ML devices show promise, but validation studies and ongoing monitoring are crucial for real-world impact.
Are there any ongoing clinical trials for validation?
Ongoing clinical trials play a crucial role in validating AI/ML-based medical devices. Here are some relevant insights:
FDA’s Role:
o The U.S. Food and Drug Administration (FDA) is actively involved in evaluating AI/ML devices for safety and efficacy1.
o The FDA clears an increasing number of AI/ML-based medical devices through the 510(k) pathway, which assesses substantial equivalence to previously cleared devices2.
Comprehensive Analysis:
o Researchers have performed comprehensive analyses of FDA-approved AI/ML-enabled medical devices, examining clearance pathways, approval timelines, regulation types, medical specialties, and recall history34.
Transparency and Guiding Principles:
o Regulatory bodies (FDA, Health Canada, MHRA) emphasize transparency for machine learning-enabled medical devices (MLMDs)5.
o Guiding principles ensure responsible development and deployment.
Remember, ongoing clinical trials provide critical evidence for the safety and effectiveness of AI/ML devices, shaping their adoption in healthcare. 🌟🔬🤖 The Promise of AI/ML in Healthcare1 New FDA Regulations on AI/ML in Medical Devices2 Comprehensive Analysis of FDA-Approved AI/ML-Enabled Medical Devices3 Transparency for Machine Learning-Enabled Medical Devices
Can you provide examples of successful trial outcomes for AI devices?
Here are some examples of successful trial outcomes for AI devices:
Trial Design Optimization:
o HINT Algorithm: Developed by the lab of Jimeng Sun, the HINT (hierarchical interaction network) algorithm predicts trial success based on drug molecules, target diseases, and patient eligibility criteria1.
o SEETrials: Intelligent Medical Objects’ SEETrials method uses OpenAI’s GPT-4 to extract safety and efficacy information from clinical trial abstracts, aiding trial designers1.
Data Analysis Automation:
o Deep Learning: AI systems enhance drug candidate prediction and safety risk assessment2.
o CliniDigest: Developed by Michael Snyder’s lab, CliniDigest summarizes records from ClinicalTrials.gov, streamlining data analysis1.
Positive Outcomes in Clinical Practice:
o Most randomized controlled trials (RCTs) evaluating AI-based medical devices in clinical practice report positive outcomes for primary endpoints3.
These examples demonstrate AI’s potential to improve trial design, data analysis, and overall clinical trial success.
Can you provide examples of failed outcomes and lessons learned from them?
Here are some examples of failed outcomes and the lessons learned from AI in clinical trials:
AI-Discovered Drug Candidates:
o BenevolentAI and Sumitomo Pharma developed AI-generated drug candidates.
o Unfortunately, these candidates failed in clinical trials due to redundancy with existing drugs1.
o Lesson Learned: AI models must consider existing treatments and avoid duplicating efforts.
Algorithm Generalization Challenges:
o In a study on schizophrenia treatments, AI algorithms predicted outcomes within specific trials but failed when applied to different patient populations2.
o Lesson Learned: AI models need robust generalization across diverse patient cohorts.
Ethical and Reproducibility Concerns:
o AI models can be biased, and their results may be hard to reproduce.
o Ensuring transparency and addressing bias are critical for reliable outcomes3.
Clinical Trial Design Optimization:
o AI tools like HINT and SEETrials aid trial design by predicting success and summarizing existing trial data34.
o Lesson Learned: AI can enhance trial efficiency and inform better designs.
In summary, while AI holds promise, rigorous validation, ethical considerations, and adaptability are essential for successful clinical trial outcomes.
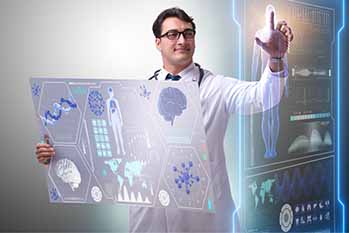
Conclusion
AI and ML are transforming medical devices, offering unprecedented insights and efficiencies. As the landscape evolves, collaboration, regulation, and ethical awareness will shape the future of healthcare.
Remember, the journey from innovation to implementation requires a collective effort, and AI/ML will continue to revolutionize patient care globally and in India.
The evolution of AI and ML in medical devices is transforming the healthcare landscape. From early diagnostic tools to advanced predictive analytics and personalized medicine, these technologies are enhancing patient care and outcomes. With significant growth in both global and Indian markets, the future of AI and ML in medical devices looks promising. Continued innovation, collaboration, and regulatory advancements will be key to unlocking the full potential of these transformative technologies.