AI Meets Sustainability
As we move deeper into 2025, sustainability has evolved from a buzzword into a core strategic priority. From climate policy to supply chain resilience, companies across sectors are under mounting pressure to cut emissions, reduce material waste, and operate with greater precision.
Nowhere is this pressure more pronounced than in manufacturing. The sector alone accounts for nearly 40% of the $40 trillion in global material waste, according to Forbes. This staggering figure underscores an urgent need: industries must adopt solutions that improve both efficiency and environmental impact.
Artificial intelligence (AI) is already playing a central role in this transformation. In 2024 alone, over $200 billion was invested globally in AI technologies, much of it aimed at industrial automation and process optimization. From predictive maintenance to supply chain forecasting, AI has proven its value in many areas. But perhaps its most critical application for sustainability lies in quality control.
The Role of Quality Control in Sustainable Manufacturing
Quality control (QC) is where efficiency and waste prevention converge. Every undetected defect, every over-scrapped part, and every line stoppage due to misclassification results in material loss, downtime, and energy waste.
Beyond improving accuracy and reducing labelling time, automated QC plays a crucial role in sustainability in general:
- Reduced Material Waste: By improving defect detection, automation can help reduce false positives and unnecessary scrapping.
- Energy Savings: Fewer reworks and shorter inspection times mean less energy consumed per unit.
- Faster Product Line Changes: Newest-Gen AI allows manufacturers to quickly adapt to new variants without weeks of retraining.
Together, these benefits support a more agile, low-waste approach to production—key pillars of any serious sustainability strategy.
Defect detection—the cornerstone of QC—plays a crucial role in minimizing this waste. But traditional systems are approaching their limits. Most AI-based inspection models today plateau at 80% defect detection accuracy, which simply isn’t good enough in industries like aerospace, automotive, or electronics manufacturing. In these contexts, anything less than 99% accuracy can mean safety risks, reputational damage, and regulatory violations.
Achieving that last jump in precision—the so-called “Last Mile Challenge”—has traditionally required enormous amounts of manually labeled data and constant retraining. It’s a resource-intensive, slow, and often unsustainable approach.
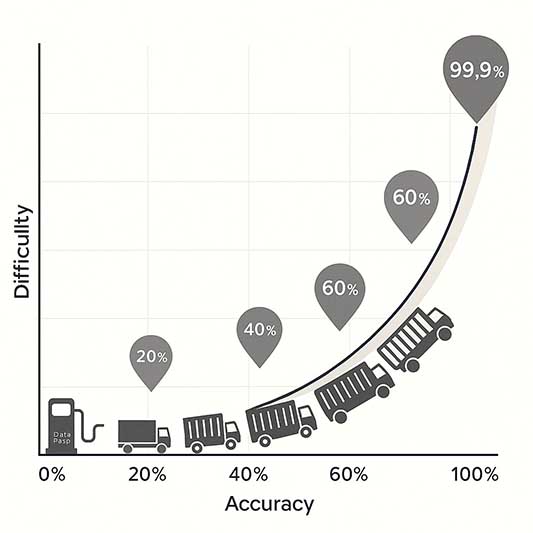
Data: The Silent Obstacle to AI Adoption
Recent research reinforces this view. According to MIT Technology Review Insights, 57% of manufacturing executives rank data quality as their primary challenge in developing AI capabilities. Among respondents from the electronics and high-tech sectors, this concern is echoed by half of all leaders surveyed.
This isn’t surprising. Manufacturing data is notoriously messy. Variability in lighting, inconsistent sensor calibration, and subtle environmental changes all introduce “noise” into image datasets.
Worse still, rare but critical defects may occur so infrequently that there simply isn’t enough real-world data to train a reliable model. And when new product lines are introduced, the
process must start all over again—collecting, labeling, and curating fresh datasets at great cost.
Industry-Wide Momentum
This issue is no longer theoretical. Tech giants have taken note. As TechCrunch (2025) reports, companies like Google have assembled dedicated synthetic data teams focused on building virtual models of the physical world. The reasoning is clear: AI cannot advance further without better training data, and synthetic data provides a scalable way to overcome real-world limitations.
Even industry voices like Elon Musk have acknowledged the trend:
“We’ve now exhausted basically the cumulative sum of human knowledge … in AI training … The only way to supplement real-world data is with synthetic data, where the AI creates training data. With synthetic data, AI will sort of grade itself and go through this process of self-learning.” (TechCrunch, 2025)
This consensus points to a future where synthetic data is not just a helpful tool—it’s an essential part of scaling AI innovation.
Synthetic Data: From Bottleneck to Breakthrough
That’s where synthetic data steps in. Instead of relying solely on data captured from the physical world, synthetic data is artificially generated to simulate real-world manufacturing conditions. It is rapidly becoming a powerful solution to the data quality and quantity issues plaguing AI-based QC systems.
Rather than waiting for defects to occur on a production line, engineers can now simulate them—on any product variant, under any lighting or texture conditions—within hours. Labels are automatically built-in, eliminating the need for tedious manual annotation.
How Synthetic Data Enhances AI-Powered Quality Inspection
Many manufacturers are increasingly turning to synthetic data to enhance defect detection and streamline quality control processes. For example, Zetamotion’s Spectron™ uses synthetic data to train AI models with as little as one initial scan of a product. This significantly reduces the need for large-scale real-world data collection and manual labeling, allowing manufacturers to deploy AI-powered inspection systems more quickly and with greater adaptability.
Wilhelm Klein, CEO of Zetamotion, explains:
“Imagine training a new QC worker. Instead of handing them thousands of labeled images, you show them a few examples, provide a defect catalog, and let them
learn from an expert. After a short while, they say, ‘Okay, I get it.’ This is a form of data synthesis—learning through context and extrapolation. Synthetic data approaches mimic this human learning process, allowing manufacturers to scale AI-powered inspection quickly and efficiently.”
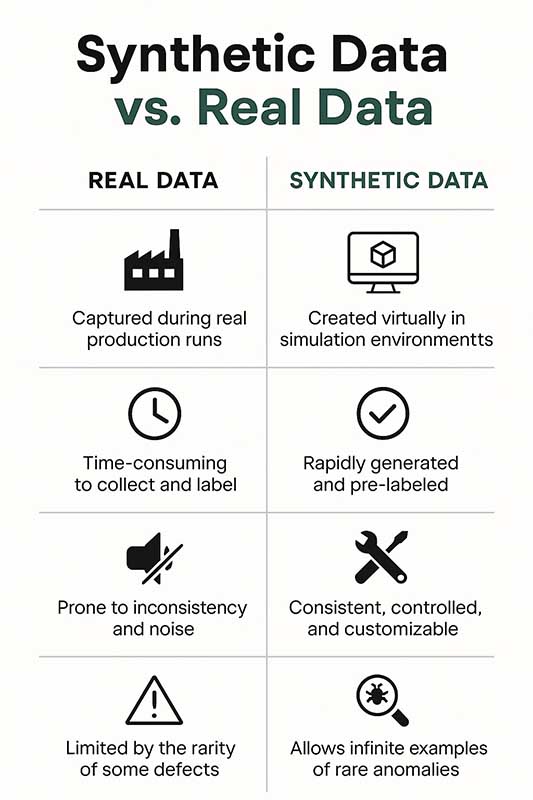
Human-in-the-Loop: Bridging Technology and Human Insight
While synthetic data and automation unlock powerful efficiencies, human expertise remains essential. Manufacturing is still, at its core, a human-centered endeavor—shaped by collaboration, adaptability, and accumulated experience.
The most effective quality control systems today integrate Human-in-the-Loop (HITL) frameworks. These systems combine the speed and consistency of AI with the judgment and intuition of experienced operators. In practice, humans validate, adjust, or override AI decisions—especially in ambiguous or edge cases—and that feedback is fed directly back into the model to improve future outcomes.
This approach doesn’t just improve accuracy. It also ensures AI evolves in step with ethical standards, operational nuance, and real-world complexity. It preserves institutional knowledge while accelerating the ability to apply it at scale.
As Dr. Wilhelm Klein explains:
“True innovation isn’t just about advancing technology—it’s about deeply understanding the needs of our partners and crafting solutions that evolve with them. Picture not a disruptive, sci-fi robot forcing its way into your processes, but rather a dedicated team handing you an exceptionally capable, intelligent magnifying glass—one that seamlessly integrates into your operations, enhancing rather than interrupting your workflow.”
In this way, HITL systems represent more than just a technical integration—they’re a philosophical one, where automation enhances human capability rather than replaces it. It’s not just smarter. It’s safer, faster, and far more future-proof.
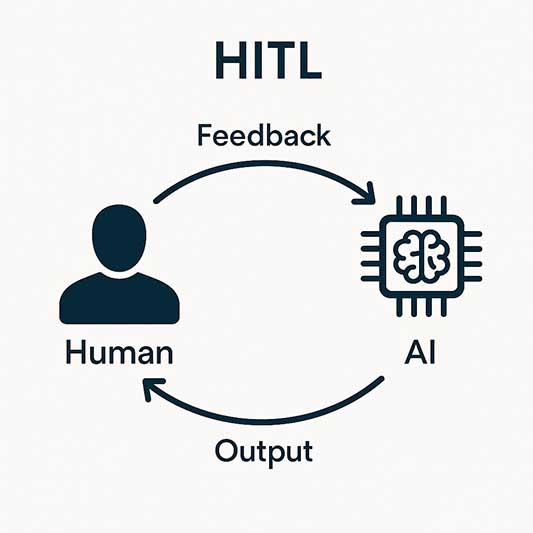
A New Era of Quality Control
In the race to build smarter, more sustainable manufacturing systems, the path forward is becoming increasingly clear: AI alone isn’t enough—data quality must come first. And synthetic data represents a transformative leap beyond the limitations of traditional methods.
Today, the barriers that once slowed down AI adoption in quality control—chief among them the need to generate and label massive datasets—are quickly disappearing. Inspection companies
can now help manufacturers by providing access to curated synthetic datasets and robust model training support, dramatically reducing time-to-implementation and lowering the need for in-house AI expertise.
This shift changes the equation entirely. Instead of months spent collecting and labeling data, manufacturers can focus on what they do best: process innovation, product refinement, and operational excellence. At the same time, synthetic data enables far more agile production environments, where inspection systems can adapt quickly to new product lines, defect types, or regulatory shifts—without restarting from zero.
As manufacturers strive to meet ambitious sustainability goals and remain competitive in a fast-moving market, the integration of synthetic data, AI, and Human-in-the-Loop (HITL)
systems offers a practical and scalable path forward. It reduces waste, accelerates deployment, and redefines what quality control can achieve in 2025 and beyond.
The tools are here. The infrastructure is growing. The need is urgent. The only question is: how soon will your production line be ready to make the leap?