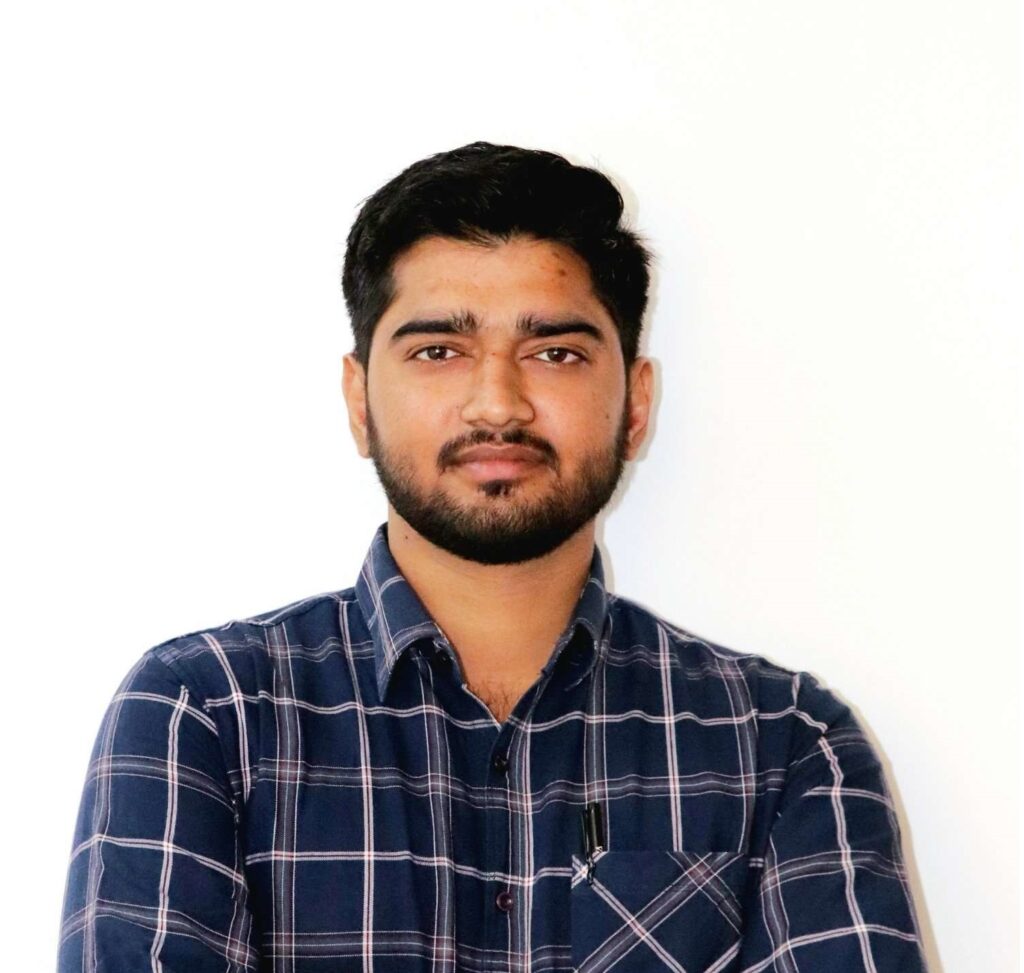
Lead Technical Consultant
LDRA
Artificial Intelligence and Machine Learning (AI/ML) are revolutionising industries, and their integration into safety-critical applications, such as aviation, healthcare, railway, and autonomous systems, is becoming transformative. However, deploying AI/ML in environments where errors could result in catastrophic outcomes requires rigorous frameworks and methodologies to ensure safety, reliability, and trustworthiness. The European Union Aviation Safety Agency (EASA) has made an attempt to address this need by developing a comprehensive concept paper. This article explores the role, challenges and regulatory approaches for deploying AI/ML in safety-critical systems, drawing insights from EASA’s concept paper.
The Role of AI/ML in Safety-Critical Applications
AI/ML excels at processing large datasets and identifying patterns, making it invaluable for applications such as predictive maintenance, anomaly detection, and decision support. In safety-critical domains, AI/ML can enhance:
- Data Processing Efficiency – Automating Complex Analyses in Real-time:
AI/ML can analyse sensor data from medical equipment and patient monitoring systems, predicting when specific components or conditions may require attention. Automating these analyses in real-time allows healthcare professionals to focus on more critical tasks and facilitate early intervention before minor issues evolve into serious health concerns, ultimately enhancing patient safety.
- Decision Support – Assisting Humans by Providing Actionable Insights:
For instance, in an aircraft cockpit, an AI/ML-based system can continuously monitor the aircraft’s performance and environmental data (e.g., weather, mechanical status, flight path, etc…). If the system detects anomalies or deviations from normal operations, it can suggest corrective actions or alert the pilot.
- Predictive Capabilities – Forecasting Potential Failures or Risks Before They Materialize:
In the railway domain, AI/ML algorithms can analyse past performance data and real-time sensor inputs from trains and railway systems to predict potential issues, such as tear of key components or track degradation. This allows maintenance teams to schedule repairs or replacements before problems cause safety risks.
- Operational Efficiency – Streamlining Tasks That Require High Precision and Adaptability:
AI/ML algorithms can be trained to carry out high-precision tasks more efficiently than humans or traditional automation systems. For example, AI/ML can optimise flight routes for fuel efficiency and safety or adjust hospital equipment settings based on real-time patient data.
Challenges in Deployment of AI/ML in Safety-Critical Systems
Despite its potential, implementing AI/ML in safety-critical environments comes with challenges.
- Trustworthiness: Ensuring AI/ML models operate reliably under all conditions.
- Explainability: Giving users comprehensible insights into the AI/ML model’s decisions.
- Data Quality: Ensuring the completeness and accuracy of training data.
- Ethics and Bias: Preventing discriminatory or unintended outcomes.
- Error Management: Mitigating risks associated with the stochastic nature of AI/ML models.
- Regulatory Compliance: Aligning AI/ML systems with stringent safety and operational standards.
Regulatory approaches for deploying AI/ML
Let’s examine how the EASA concept paper addresses some of the above challenges by building trustworthiness, explainability, regulatory implications, and future directions.
Building Trustworthy AI/ML: The Five Pillars
EASA’s framework1 emphasises five building blocks essential for developing trustworthy AI/ML, which are mapped to the seven gears of the Assessment List for Trustworthy AI published by the EU Commission2 – High-Level Expert Group on ML.
- Trustworthiness Analysis:
- Assess safety, security, and ethical considerations.
- Conduct thorough evaluations to characterise the AI/ML application and its operational context.
- AI Assurance:
- Extend traditional development assurance methods to address AI/ML-specific challenges.
- Focus on data quality, robustness, and the generalizability of trained models.
- Ensure continuous monitoring and post-operational safety assessments.
- Human Factors:
- Develop user-centric designs that foster collaboration between humans and AI/ML systems.
- Ensure operational explainability to enhance user trust and facilitate effective decision-making.
- Risk Mitigation:
- Address residual risks through robust error management systems.
- Enhance transparency to minimise uncertainties associated with AI/ML models.
- Organisations:
- Organisations must update processes to ensure AI/ML trustworthiness, including managing security risks and conducting continuous safety assessments throughout the AI/ML lifecycle.
- They should establish ethical oversight, provide AI-specific training, and adapt certification and risk management processes to ensure the safety and reliability of AI/ML systems.
Explainability: A Cornerstone of AI/ML Safety
Explainability is crucial for user trust, especially in safety-critical domains. EASA categorises it into two aspects,
- From the perspective of the end-user, i.e. operational explainability and
- From the perspective of software developer, i.e. development explainability
additionally, we must consider explainability from the perspective of External entities3 like regulatory agencies.
All of these profiles never expect the same kind of explanation; usually, end-users do not want to get clarity about the internal details of the model, which are very important for a developer to understand the relationship between input and output to validate the precision and accuracy of the AI/ML model.
- Explanations in AI/ML models: While AI/ML models are harder to explain due to their complexity, explanations are crucial for understanding the relationship between inputs and outputs, ensuring trust, identifying biases and improving model accountability.
- Certification of AI/ML Systems: Explanations are essential for certification, ensuring that AI/ML systems comply with safety and legal standards and helping human operators supervise and manage autonomous systems, particularly in safety-critical contexts.
- Challenges for Explainability: Key challenges include ensuring the interpretability of explanations, providing sufficient information for users, developers and regulators, defining explainability metrics, and investigating incidents to understand the causes behind AI/ML model decisions.
Regulatory Implications and Future Directions
The integration of AI/ML models into existing safety frameworks is essential to improve the efficiency of existing systems and to implement requirements that are difficult to meet with traditional approaches in safety-critical applications. Aligning with broader AI regulations, such as those from the EASA, provides preliminary guidance for advanced AI systems, and continuous collaboration among stakeholders is crucial to refine and establish industry standards.
While AI/ML offers substantial potential in safety-critical applications, its integration with traditional systems requires rigorous validation and verification of systems to meet safety and compliance standards. LDRA’s comprehensive tool suite helps identify errors and ensures code quality and reliability of non-AI/ML components, aligning with industry standards for functional safety (such as DO-178C in Aviation). LDRA, therefore, plays a crucial role in maintaining safety and reliability in systems that integrate with AI/ML by validating their supporting software infrastructure and ensuring compliance with safety-critical regulations.
Conclusion
AI/ML offers transformative potential for safety-critical applications, but its integration demands rigorous frameworks to address reliability, transparency, and ethical concerns. EASA’s concept paper provides a robust starting point, emphasising trustworthiness, human and AI/ML system collaboration, and risk management. As AI and ML technologies advance, it is crucial to continuously refine these principles and regulations to fully leverage their potential while prioritising safety.
About Author:
Shubham Pratap Singh is a Lead Technical Consultant at LDRA with over seven years of experience in embedded systems. He has spent two years as an Embedded Developer and the past five years specialising in Embedded Safety and Security, assisting clients with static analysis, dynamic analysis, and unit testing. He holds an Electronics and Communication Engineering (ECE) degree from Dr. A.P.J. Abdul Kalam Technical University.
References
- European Union Aviation Safety Agency (EASA). Concept Paper: Guidance for Level 1 & 2 Machine Learning Applications, Issue 02.
- EU Commission (2021). Proposal for a Regulation of the European Parliament and of the Council laying down harmonised rules on artificial intelligence (Artificial Intelligence Act).
- DEEL Certification Workgroup (2021). White Paper on Explainability in AI Systems.