Introduction
The electronics manufacturing industry market is only expanding, according to IPC, the rising demand for electronic devices across multiple industries will see the market grow from about $534 billion in 2023 to $856 billion in 2030. Integrating artificial intelligence (AI) is crucial for the development of the industry. According to the Taiwanese Market Intelligence & Consulting Institute (MIC), nearly 65% of companies in Taiwan have invested in AI, and up to 57% have adopted AI-enabled AOI solutions to enhance defect detection through machine learning. This article explores the latest advancements in AI and the current landscape of AOI, shedding light on how this transformative technology is reshaping quality control in electronics manufacturing.
Current PCBA Challenges
The complexity of modern printed circuit boards (PCBs) presents significant challenges in the electronics manufacturing industry. Traditional Automated Optical Inspection (AOI) systems often struggle with:
- High Component Densities and Fine Pitches: As PCBs become more complex, the precision required to inspect them increases, leading to potential inaccuracies, higher false calls and escapes.
- Labor Shortages: The lack of skilled labor exacerbates the challenge, causing higher costs, increased rework, and shipment delays. According to Bloomberg, the US risks a technician shortage reaching 90,000 by 2030. Natcast’s forecast found that out of 238,000 jobs the industry is projected to create by 2030, the semiconductor industry will be unable to fill more than 67,000 positions.
- High False Call Rates: Traditional systems often generate many false positives, reducing production efficiency and increasing unnecessary rework.
- Complex Programming: Setting up AOI systems for high-mix, low-volume production requires significant expertise and time, creating a bottleneck in the manufacturing process.
AI Technologies Addressing Inspection Challenges
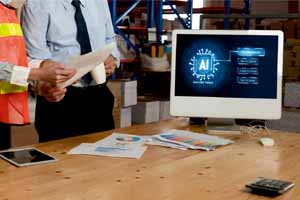
AI is at the forefront of addressing these challenges, especially machine learning and deep learning. By leveraging neural networks trained on vast datasets of PCB images, AI-powered inspection systems can analyze and interpret visual data with unprecedented accuracy and speed. Unlike rule-based systems, AI models continuously improve performance and adapt to new defect types and component variations.
The benefits of integrating AI into AOI processes are substantial. Early adopters have reported significant reductions in false call rates, with some achieving up to 90% fewer false positives than traditional systems. This improvement increases production efficiency and reduces the strain on operators verifying potential defects. For example, a leading optical inspection equipment vendor reported a 50% reduction in operator workload, an 80% improvement in cycle time, and minimized human escape to only 0.05%. These significant benefits underscore the positive impact of AI integration.
Moreover, AI is revolutionizing the programming and setup of AOI systems. Machine learning algorithms assist in creating inspection recipes, dramatically reducing the time and expertise required to program new products. Some AI-powered systems can generate initial inspection parameters automatically, requiring only minor adjustments from operators. This capability is valuable in high-mix production environments, where frequent changeovers have historically been challenging. Integrating 3D measurement data with AI analysis enables more accurate inspection of component height, coplanarity, and solder joint quality. The efficiency gains from these advancements are truly promising.
Implementation Challenges and Solutions
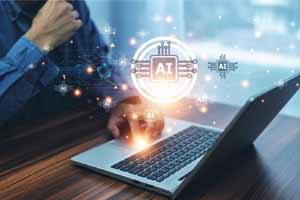
Implementing AI capabilities is not without its challenges. Key considerations include GPU and software version requirements, data quality, and cybersecurity. Synthetic data generated by computer simulations can supplement real-world data, reducing training time and costs. However, vendors’ requirements for model training can vary significantly, with some needing fewer than 50 images for initial training.
Data safety and cybersecurity pose additional concerns. As organizations increasingly rely on cloud-based solutions for data storage and processing, they face the risk of cyberattacks compromising data security and privacy. To mitigate this risk, large EMS and data-conscious manufacturers often opt to install AI servers on-site, ensuring that data remains within the premises. Major equipment vendors offer alternative solutions for data safety and AI server location.
Best practices for implementing AI in AOI are emerging from early adopters and industry leaders. Successful implementations typically involve cross-functional collaboration between quality control, IT, and production teams. A phased approach, starting with pilot projects and gradually expanding, allows organizations to learn and adapt. Continuous monitoring and refinement of AI models are essential to ensure ongoing performance improvements and adaptation to new products or processes.
Future Impact of AI in EMS and AOI
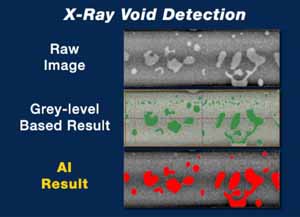
Looking to the future, PCBs, programming, and yield management are becoming more complex. The labor force cannot keep up with advancements while remaining cost-effective. AI’s biggest impact will be addressing the labor shortage of skilled operators. AI Repair Stations will become an industry standard as AI inspection surpasses manual buy-off and offers lower false-call rates.
Case studies from the industry highlight the transformative potential of AI in AOI. One major electronics manufacturer reported a 50% reduction in overall defect escape rates after implementing an AI-powered inspection system, coupled with a 30% increase in production throughput due to reduced false calls and faster programming. Another case study demonstrated how a high-mix, low-volume producer could reduce new product introduction times by 60% through AI-assisted AOI programming. In a U.S.-based plant evaluation, an AI platform identified errors in roughly 5% of boards during a one-month evaluation period, resulting in projected cost savings exceeding $100,000 per production line annually. The savings came from reduced rework, prevented scrap, and improved first-pass yield.
TRI AI-powered Solutions
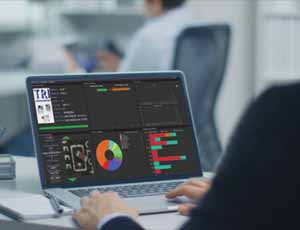
With over 35 years of experience in the field, Test Research, Inc. (TRI), the leading test and inspection equipment provider for the SMT and Advanced Packaging Industry, has developed multiple AI-powered solutions. TRI’s first introduction to the market with AI-related applications was over five years ago with the deep learning OCR. Other developed AI solutions are AI Smart Programming for faster setup, AI repair station for optimizing buy-off, AI detection for defect detection on the inspection equipment, and more.
TRI AI Smart Programming facilitates predictive decision-making like an expert or experienced operator, reducing programming time, downtime, and operator training. Traditional programming of 718 components would take around 60 minutes; AI programming reduces this to just 9 minutes, an 85% improvement.
Thanks to TRI’s AI verification advancements, the detection rate of components has improved significantly. The AI detection accuracy for general chip defects now exceeds 99%. Specific improvements include a detection rate of over 95% for components such as OSC, MLD, SOD, SOT23, RNET, and CNET. X-ray void detection with AI implementation improves the first pass yield rate (FPY) from 85% to 98%. For complex components like the Paladin connector, AI classification reduces the false case rate from approximately 25,000 ppm to around 3,000 ppm, an 88% improvement. For Optical Character Verification (OCV), the FPY rate has improved from 70% to 90% when using AI methods compared to conventional OCV. Additionally, while third-party AI libraries can only achieve a character recognition rate of 90%, TRI’s AI achieves over 99.5% accuracy at a higher speed.
AI Verify Host is TRI’s answer to AI Buy-Off in repair stations. It is an AI repair station that reduces the need for manual re-inspection and lowers operational costs. Typically, repair station operators perform poorly, and training is not cost-effective. The AI-powered repair station can continuously operate efficiently, outperforming operators while lowering false calls and providing real-time data analytics of the inspection status.
TRI also has its proprietary Training Tool to generate AI models from existing inspection data. The tool includes a built-in AI labeling tool and supports classification, segmentation, OCR, detection tools, continued training, heat maps, and more. The model can be deployed in TRI’s Optical Inspection (SPI, AOI, and AXI) software, AI-Verify Host (buy-off repair station), or on the AI Station. The latter supports multiple AOIs and smart scheduling, reducing AI hardware costs and improving resource management.
Conclusion
Integrating AI-driven inspection for the electronics manufacturing industry represents a new frontier in quality control capabilities. The technology promises to address longstanding challenges in accuracy, efficiency, and adaptability, enabling manufacturers to keep pace with the increasing complexity of modern electronics. While challenges in implementation remain, the potential benefits in terms of improved quality, reduced costs, and increased productivity are compelling.
AI plays a central role in shaping the future of electronics manufacturing. The industry must embrace this technology, investing in research, development, and workforce training to fully realize its potential. Collaboration between technology providers, manufacturers, and researchers will be key to overcoming current limitations and pushing the boundaries of what is possible in automated inspection.
As the technology evolves, we expect to see even more sophisticated applications, from AI-driven design for manufacturability (DFM) analysis to fully autonomous inspection and repair systems. Integrating AI with other emerging technologies, such as augmented reality for operator assistance and advanced robotics for automated rework, promises to further revolutionize the PCBA manufacturing landscape. Ultimately, the successful adoption of AI in optical inspection will be a critical factor in determining competitiveness in the rapidly evolving electronics manufacturing industry. Those who embrace and master this technology will be well-positioned to deliver higher-quality products, respond more quickly to market demands, and operate more efficiently than ever. As we move forward, AI’s continued advancement and refinement in AOI will undoubtedly play a pivotal role in shaping the future of electronics production, driving innovation, and setting new standards for quality and reliability.