As generative artificial intelligence (AI) adoption grows at record-setting speeds1 and computing demands increase2, hybrid processing is more important than ever. But just like traditional computing evolved from mainframes and thin clients to today’s mix of cloud and edge devices, AI processing must be distributed between the cloud and devices for AI to scale and reach its full potential.
A hybrid AI architecture distributes and coordinates AI workloads among cloud and edge devices, rather than processing in the cloud alone. The cloud and edge devices — smartphones, cars, personal computers, and Internet of Things (IoT) devices — work together to deliver more powerful, efficient and highly optimized AI.
The main motivation is cost savings. For instance, generative AI-based search cost per query is estimated to increase by 10 times compared to traditional search methods3 — and this is just one of many generative AI applications.
Hybrid AI will allow generative AI developers and providers to take advantage of the compute capabilities available in edge devices to reduce costs. A hybrid AI architecture (or running AI on-device alone) offers the additional benefits of performance, personalization, privacy and security at a global scale.
These architectures can have different offload options to distribute processing among cloud and devices depending on factors such as model and query complexity. For example, if the model size, prompt and generation length is less than a certain threshold and provides acceptable accuracy, inference can run completely on the device. If the task is more complex, the model can run across cloud and devices.
Hybrid AI even allows for devices and cloud to run models concurrently — with devices running light versions of the model while the cloud processes multiple tokens of the full model in parallel and corrects the device answers if needed.
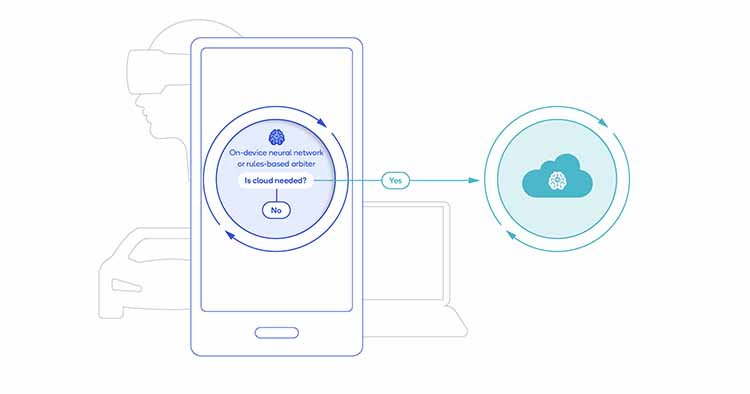
Scaling generative AI with edge devices
The potential of hybrid AI grows further as powerful generative AI models become smaller while on-device processing capabilities continue to improve. AI models with more than 1 billion parameters are already running on phones with performance and accuracy levels similar to those of the cloud, and models with 10 billion parameters or more are slated to run on devices in the near future.
The hybrid AI approach is applicable to virtually all generative AI applications and device segments — including phones, laptops, extended reality headsets, cars and IoT. The approach is crucial for generative AI to scale and meet enterprise and consumer needs globally. We truly believe that the future of AI is hybrid. Read our whitepaper to learn more.