Today, more than ever, all branches of industry are striving to combine short production times and high quality with the lowest possible cost. Production downtime due to unplanned maintenance work is just as much a liability as parts that are replaced unnecessarily or too early. How can predictive maintenance help to optimize maintenance schedules, maximize asset lifetime, and minimize physical wear?
By Luca Villa and Manuel Hollfelder, Infineon Technologies
Not too long ago, assets were maintained reactively, i.e. they were exchanged or repaired when they stopped functioning. Such an approach maximizes the utilization of the asset as it is used until its end-of-life (EoL). This, however, means that the company faces catastrophic unplanned downtime. For instance, production downtime has a negative impact on the continuity of industrial operations and the company’s ability to meet productivity targets.
Because of the disadvantages of reactive maintenance, industrial companies have adopted a preventive approach to asset management, also called preventive or periodic maintenance. In this paradigm, equipment is maintained before its EoL to prevent unplanned downtime. So, the nominal life of the equipment as specified by the OEM (Original Equipment Manufacturer) is taken into account.
Periodic maintenance allows industrial companies to ensure the continuity of their processes, as maintenance can be scheduled at times when operations are not disrupted. Still, periodic maintenance is far from being the best possible asset management model. The reason is that assets are not being used to their fullest potential and the company’s overall equipment efficiency (OEE) is suboptimal.
To mitigate these limits, companies are implementing condition monitoring and condition-based maintenance (CBM) of assets. Instead of relying on predetermined values of the nominal life cycle of the asset to schedule maintenance, CBM uses the actual condition of the asset to determine whether it should be maintained, replaced, or repaired (Figure 1).
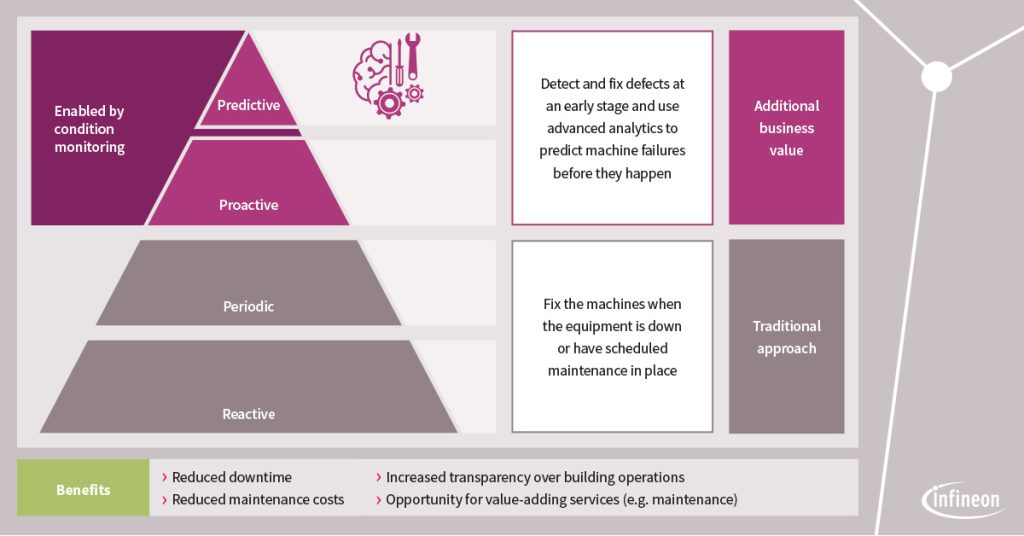
For this purpose, CBM analyzes data reflecting the actual physical condition of the asset, e.g. information on the operating vibrations and temperature of the asset, as well as data on the energy consumption or oil analysis of the asset. CBM allows maintenance engineers to identify issues with the assets (e.g. failures, wear) in an accurate and timely fashion. As such, it can also enable fast and effective maintenance processes.
Predictive maintenance (PdM) is one of the most prominent and advanced cases of CBM. It analyzes information about the condition of the asset to accurately predict its Remaining Useful Life (RUL). Specifically, RUL predictions are derived based on predictive analytics algorithms via sensor data, such as data from vibration, temperature, acoustic, and ultrasonic sensors, as well as thermal images. RUL estimation is a foundation for optimal scheduling of the assets’ maintenance, which leads to the best possible OEE. In this way, PdM delivers tangible benefits to enterprises, including improved utilization of assets, as well as high-quality and cost-effective operations.
Predictive maintenance is currently considered one of the most prominent use cases of the fourth industrial revolution (Industry 4.0) since it is relevant to a wide array of industrial sectors and their critical infrastructure including manufacturing, energy (Figure 2), oil & gas, mining, smart cities, smart buildings, and facilities management. Specifically, PdM is extensively used in industrial automation applications in manufacturing shopfloor, oil refineries, and energy plants.
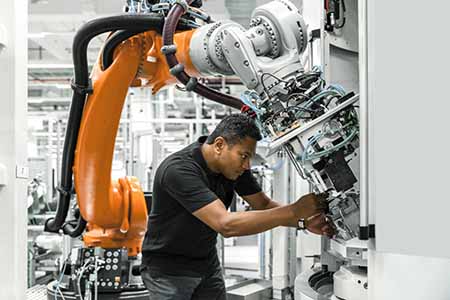
The technology behind predictive maintenance
The development and deployment of CBM and PdM systems hinge on the integration of high-fidelity sensing systems with state-of-the-art digital technologies. In particular, a PdM system comprises the following technological elements:
- Sensors and Internet-of-Things (IoT) Devices: Sensors and other internet-connected devices, such as IoT-enabled embedded systems, allow the acquisition of data about the condition of the physical asset. In most cases, IoT middleware is used to automate the data collection process. As already outlined, different types of sensors can be used to identify the condition and the status of the asset. Moreover, there are PdM applications that combine and fuse information from multiple sensing modalities to achieve greater accuracy.
- Big Data Management: In most cases, PdM applications leverage massive volumes of data about physical assets to identify and assess their condition. In several cases, sensor data is analyzed with data from enterprise systems such as ERP (Enterprise Resource Planning) and CMMS (Computerized Maintenance Management) systems. Moreover, sensor information features high ingestion rates and tends to comprise noisy and uncertain data. Therefore, most PdM applications must process big data that features data of high volume, velocity, and veracity. In this direction, PdM deployments comprise big data management systems, including historian databases and data lakes.
- Cloud Computing and Edge Computing: Nowadays, many PdM systems are deployed within cloud computing infrastructures. This enables predictive maintenance systems to benefit from the capacity, scalability, and quality of service of the cloud. Specifically, cloud infrastructures enable PdM developers and deployers to flexibly access the storage and computing resources needed to manage and analyze maintenance-related big data. There are also cases where condition monitoring and predictive maintenance insights must be analyzed in real time. In such cases, edge computing and edge devices are usually deployed in conjunction with the cloud infrastructure.
- Big Data Analytics & Machine Learning: Most PdM applications hinge on credible predictions about the RUL and other critical conditions (e.g. failure modes) of the asset. In this direction, they employ predictive analytics such as regression techniques. Moreover, machine learning techniques are commonly used to predict the asset’s status and provide prescriptive recommendations about how and when to maintain it.
- Visualization: PdM insights must be visualized in ergonomic and user-friendly ways to enable technicians and maintenance engineers to perform their tasks (Figure 3) effectively. In this direction, information about failure modes and RUL estimations are visualized in dashboards and charts. There are also cases where maintenance instructions and recommendations are displayed as Augmented Reality (AR) cyber-representations over the asset to facilitate workers in maintenance and repair tasks.
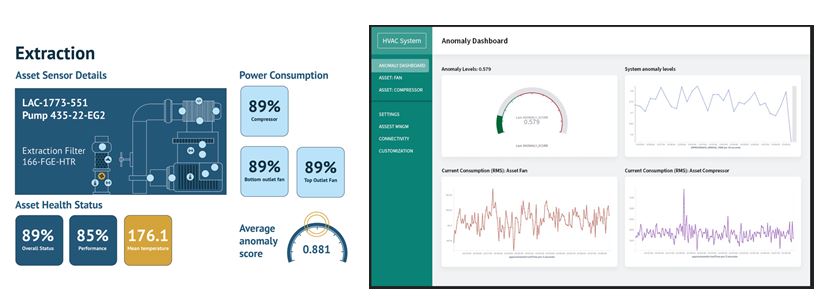
Integrating these modules and technology building blocks into end-to-end solutions may pose a challenge because the building blocks mentioned above are not always compatible with each other. For example, there is usually a mismatch between the data models generated by the hardware and the models used by the machine learning algorithms. In this respect, companies get better value by using PdM platforms and services that mitigate this heterogeneity and ensure the interoperability of the miscellaneous modules.
Regarding hardware, there are platforms and maintenance kits that integrate various sensors in one hardware box. Practically, the development of end-to-end solutions is also challenging from an economic point of view. This is because an integrated end-to-end solution involves licensing and using elements from different vendors, including hardware vendors, cloud infrastructure providers, and software/analytics vendors.
Predictive maintenance applied
With the above technologies, it is possible today to develop a wide range of PdM-based applications that help industrial companies improve the quality, timeliness, and cost-effectiveness of their operations. Here are some popular use cases:
- Intelligent Asset Management based on Smart Failure Mode Effects and Criticality Analysis (FMECA): FMECA is a popular approach for assessing the failure risks of physical assets with a view to preventing problems and malfunctions. It is based on a data-driven estimation of failure chains, including the failure mode, the effects of the failure, and its causes. Predictive maintenance systems can be used to predict and anticipate failure modes for specific assets while linking them with their potential causes. Hence, PdM deployments enable maintenance engineers to improve the accuracy and predictive power of their FMECA processes.
- Quality Management and Zero Defects Manufacturing: Malfunctioning assets are one of the most prominent causes of defects and production quality issues in manufacturing. For instance, an asset that does not function well is likely to cause wear that leads to defective products. PdM systems can therefore be used to proactively anticipate asset issues and to trigger actions that prevent them from happening. This is a foundation for improving production processes towards quality excellence. Moreover, PdM systems are among the main enablers of the vision of Zero Defect Manufacturing (ZDM), which employs a variety of preventive and predictive measures to ensure that no defective products are produced on an industrial site.
- Remote Maintenance using Augmented Reality: PdM can help reduce maintenance times when used with AR-based remote maintenance (Figure 4). In this paradigm, a maintenance engineer of the OEM of the assets provides remote service or repair instructions to an on-site technician. The instructions are planned according to information about the asset’s status that is provided by a condition monitoring and/or PdM system. This process obviates the need for travel to the site and accelerates the maintenance and repair process.
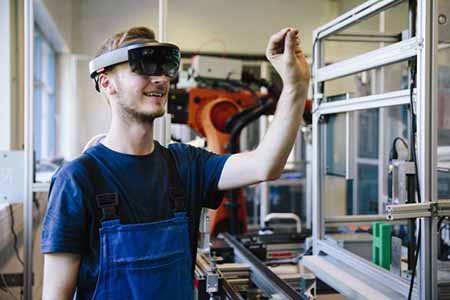
Predictive maintenance solutions by Infineon
As a leading semiconductor manufacturer, Infineon offers several cutting-edge predictive maintenance solutions, including hardware, software, simulation, and analytics offerings. The XENSIVâ„¢ predictive maintenance evaluation kit, for example, enables the development of end-to-end sensor-enabled condition monitoring and predictive maintenance solutions. It allows the general development of popular use cases such as condition monitoring and predictive maintenance, e.g. for pumps and motors in industrial automation, or compressors and fans in HVAC equipment. In this direction, the kit leverages Infineon’s broad XENSIVâ„¢ sensor portfolio along with Infineon’s broad microcontroller portfolio. This sensor portfolio supports the collection of a broad range of physical parameters from different assets, including:
- Air flow measurement at the compressor based on the XENSIVâ„¢ DPS368 barometric pressure sensor
- Current measurement at the fan and compressor based on the XENSIVâ„¢ TLI4971 current sensor
- Position sensing of the motor with XENSIVâ„¢ TLI493D-A2B6 3D magnetic sensor
- Sound anomaly detection in the unit with XENSIVâ„¢ MEMS microphones
- Linear vibration measurement with XENSIVâ„¢ TLE4997E linear Hall sensor
- Opened and closed lid detection with XENSIVâ„¢ Hall sensors
- Speed and direction measurement with XENSIVâ„¢ TLI4966G double Hall sensor
- Data processing with XMCâ„¢ 4700 or PSoCâ„¢ 6 microcontrollers
- Secured connection and authentication with OPTIGAâ„¢ Trust M security solutions
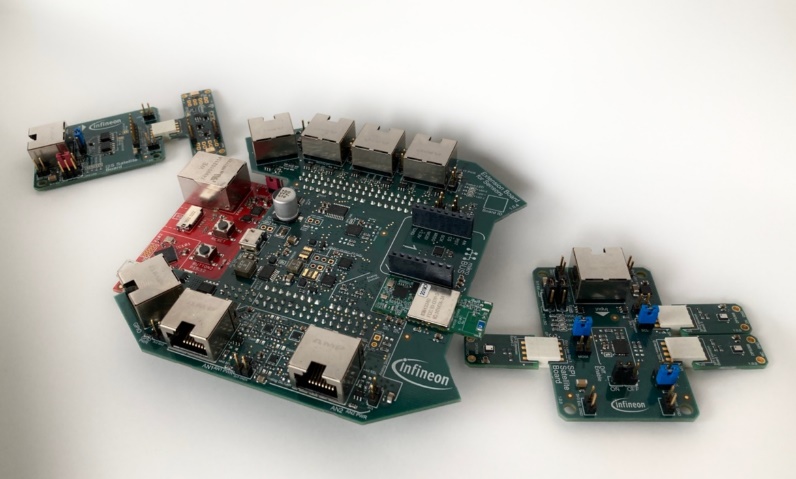
Industrial organizations can implement various predictive maintenance scenarios based on these sensors and the collected data. For example, in the case of Quality Management and Zero Defects Manufacturing, the XENSIVâ„¢ sensor portfolio enables the collection of condition data about various elements of the units of manufacturing devices such as filters, compressors, or motors. Furthermore, the XENSIVâ„¢ predictive maintenance evaluation kit facilitates the management of sensor data in the cloud, and analysis of this data utilizing advanced predictive analytics and machine learning techniques. But also, machine learning at the edge is enabled through Infineon’s ModusToolboxâ„¢ and the global partner ecosystem.
IPOSIM Lifetime Estimation service
Infineon provides a novel Lifetime Estimation service for industrial assets. It is an enterprise-scale 24/7 service that leverages lifetime estimation algorithms and Infineon’s expertise to facilitate the design process. Customers of this service can therefore benefit from Infineon’s expertise in power electronics to simplify and accelerate their design processes.
The IPOSIM service offers access to three different simulation types, including:
- Steady-state simulation for power and thermal calculation of a single cycle
- Load cycle simulation for power and thermal calculation of multiple operating points
- Lifetime estimation for expected lifetime calculation based on chosen parameters
The service is accessible through a user-friendly Graphical User Interface (GUI). The GUI guides designers in a step-by-step process through the simulation with power devices. Overall, the IPOSIM service facilitates the selection of the most suitable high-power products for a given set of application requirements. Moreover, it helps to reduce development costs, to optimize product sizing, reduce the bill of materials (BOM), and accelerate time-to-market.
Industrial Analytics AI services
Infineon-owned Industrial Analytics provides AI (Artificial Intelligence)-based analytics services for intelligent asset management applications. Specifically, the company offers access to advanced analytics services for plant monitoring and timely detection of assets’ degradation based on various sensing modalities such as vibration sensing. The analytics solutions include predictive maintenance based on predictive analytics functions, as well as the provision of prescriptive recommendations about how to best maintain, service, and repair industrial assets. The offered services take advantage of Industrial Analytics’ AI expertise and Infineon’s in-depth semiconductor industry knowledge.
Conclusion
In our fast-moving times, it is becoming increasingly important that industrial assets are available with the least possible downtime while operating as cost-effectively as possible. This can best be achieved with a condition-based monitoring and predictive maintenance-based approach, which helps to optimize maintenance schedules, maximize asset lifetime, and minimize physical wear.
Infineon’s solutions span all the crucial elements to implement end-to-end predictive maintenance: From reliable sensing to advanced AI-based analytics. The solutions are scalable, integrated, and easy to deploy. This helps industrial enterprises to accelerate the development and deployment of a wide range of condition monitoring and predictive maintenance in a variety of configurations.
Visit www.infineon.com/predictivemaintenance for demo videos and more information.
Luca Villa
is Business Development Rep Emerging Applications for Building Automation in the Power and Sensor Systems division, where he is responsible for commercial business development for building automation such as predictive maintenance and Power over Ethernet. He has extensive experience in commercial roles in the semiconductor industry, starting his career in Arrow Electronics as an account manager in Italy before proceeding to his current role at Infineon. During his time at Arrow, he gained experience in different international projects focused on predictive maintenance, and his current role has enabled him to further focus on this application. He joined Infineon in June 2022 and holds a Master’s Degree in International Business Administration from the University of Bergamo, Italy.
Manuel Hollfelder
is Senior Manager Emerging Applications and he leads the Emerging Applications Architecture and Engineering team in the Power & Sensor Systems (PSS) division. His role involves working together with his team on new and emerging applications as well as driving the technical business development. Moreover, he is also working on condition monitoring and predictive maintenance enablement for focus applications, e.g. for HVAC or power systems. He joined Infineon in 2016 and holds a Master’s Degree in Theoretical Physics from the University of Bayreuth, Germany, as well as an MBA from the Collège des Ingénieurs in Paris.