Semiconductor chip design is facing new challenges with complex architectures and a growing need for performance and energy efficiency. Traditional methods are struggling to keep up, which is where Artificial Intelligence (AI) comes in. AI is not just an addition; it’s a game-changer in how we approach chip design. Designs of semiconductor chips are getting more intricate, making it tough for traditional methods to handle. These designs demand careful attention, and the old ways of doing things are struggling to keep pace. Achieving both high performance and energy efficiency has become tricky, and the usual methods are starting to feel the strain. In this challenging environment, Artificial Intelligence (AI) emerges as a revolutionary force. It’s not just another tool; it’s changing the very nature of chip design.
Optimizing chip designs with AI
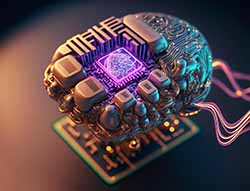
When it comes to improving chip designs, AI-powered neural networks lead the way. These networks, inspired by the human brain, navigate complex designs efficiently. Trained on massive datasets, they can recognize patterns and suggest optimal configurations, speeding up the design process and introducing innovative solutions. Alongside neural networks, genetic algorithms take cues from nature to optimize chip designs. They continuously refine solutions, often discovering new configurations that traditional methods might miss.
AI introduces a new era of automation in chip design, mitigating manual efforts and accelerating design phases. AI streamlines the layout generation process, reducing the margin for human error and expediting design timelines. In Synthesis and Optimization, AI-driven tools automate complex tasks, ensuring designs meet and often surpass performance criteria.
Designing the chips with AI
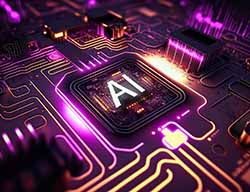
In semiconductor design, RTL to GDSII represents the transition from a high-level logical representation of the chip to its physical realization. AI integration in this process brings better optimization, efficiency, and precision in various key stages.
Traditionally, synthesis involves converting the RTL design into a gate-level netlist, a critical step in defining the logical structure of the chip. With AI integration, synthesis becomes more adaptive and optimized. Machine learning algorithms analyze patterns in previous synthesis processes, identifying efficient pathways and optimizing the logic to enhance performance. This adaptability ensures that the chip’s logical representation is not just a translation but an optimized blueprint for the next stages.
As energy efficiency becomes increasingly important in low-power synthesis where AI’s adaptive nature, excels in optimizing power consumption. Machine learning models analyze the power intent specified in the design, adapting synthesis strategies to minimize power usage while maintaining performance. This integration ensures that the chip not only meets performance criteria but also aligns with power efficiency standards. Moving into the Place and Route stage, traditional methods face challenges in achieving the delicate balance between minimizing area, reducing power consumption, and meeting timing constraints. AI-driven optimization considers a multitude of factors simultaneously, adapting the placement of logical elements and routing connections to achieve an optimal balance. This adaptability ensures a more efficient use of silicon real estate and improved overall performance.
AI also enhances the static timing analysis process by predicting and mitigating timing violations more accurately. Machine learning models analyze vast datasets of timing violations and resolutions, learning from past experiences to predict potential issues in the current design. This proactive approach ensures that timing constraints are met, minimizing the need for iterative adjustments.
AI-enhanced verification and testing
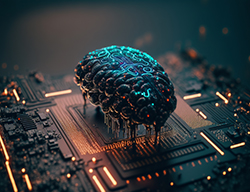
Ensuring the reliability of semiconductor chips is critical for their effective performance. AI significantly enhances the verification and testing processes. AI algorithms are equipped with advanced machine-learning capabilities and excel at dynamic pattern recognition. Trained on extensive datasets, these algorithms learn the variation in chip behavior, identifying potential weaknesses in designs that may be challenging to detect for traditional verification approaches. This dynamic adaptability allows for the early detection of issues, enabling corrective measures before mass production.
Also, AI-driven simulation tools revolutionize traditional methodologies by leveraging intricate algorithms to provide more accurate predictions. These tools, powered by AI, create a bridge between simulated environments and real-world scenarios. By incorporating a deep understanding of the chip’s behavior gained through extensive training, AI-driven simulations offer insights that closely align with actual performance expectations. This reduces the gap between simulated results and real-world outcomes, providing a more reliable basis for decision-making.
Challenges in AI-driven chip design
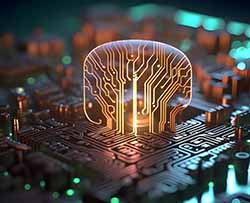
Designing semiconductor chips with AI introduces a bring many challenges that affect various stages of the design process.
- Data quality: AI models rely heavily on vast and high-quality datasets for effective learning. In semiconductor chip design, obtaining comprehensive datasets that accurately represent the complexities of different design scenarios can be challenging. Insufficient or inaccurate data may lead to biased models, suboptimal design suggestions, and increased risk of errors in the final chip
- Complex algorithms: Developing AI algorithms that are robust enough to handle diverse and complex design scenarios is a significant challenge. AI models need to adapt to dynamic environments and unexpected challenges during the design process. These algorithms may struggle to provide accurate predictions or recommendations, especially when faced with design complexities or variations
- Interpretability: AI models often operate as “black boxes,” making it challenging to interpret and explain their decision-making processes. Understanding why a particular design decision was recommended or made is crucial for validation and acceptance. Lack of interpretability can hinder the trustworthiness of AI-driven design decisions, making it difficult for designers to comprehend and validate the choices made by the AI models
- Tool integration: Integrating AI methodologies seamlessly into existing Electronic Design Automation (EDA) tools can be challenging. Compatibility issues and the need for collaborative tool development may hinder the smooth incorporation of AI into the design workflow. Difficulty in tool integration may slow down the adoption of AI in chip design and limit its effectiveness in improving existing design processes
Future trends in AI and Semiconductor chip design
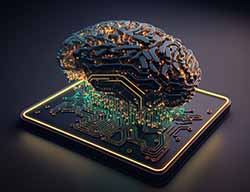
The future trends of AI in semiconductor design promise a landscape where innovation, efficiency, and adaptability converge to redefine the possibilities of chip development. As AI continues to mature, its integration into semiconductor design workflows is expected to catalyze advancements that will shape the trajectory of the electronics industry. This collaboration between human ingenuity and AI-driven methodologies positions the semiconductor industry at the forefront of technological innovation, unlocking new frontiers in chip design, and paving the way for a future where AI is an indispensable ally in the creation of cutting-edge semiconductor technologies.
However, AI is also making inroads into semiconductor manufacturing. From optimizing production processes to predicting equipment maintenance needs, AI is revolutionizing the manufacturing landscape. Predictive maintenance, yield optimization, and defect detection are areas where AI is contributing to increased efficiency and reduced costs in semiconductor fabrication plants.
In conclusion, the integration of AI into semiconductor design processes has ushered in a new era of efficiency, innovation, and reliability. MosChip’s expertise in RTL design, design verification, physical design, and analog layout, combined with the power of AI, exemplifies the industry’s commitment to staying at the forefront of technological advancements. As we look to the future, the synergy between human creativity and AI-driven optimization promises to unlock even greater possibilities in semiconductor design and manufacturing. As technology continues to advance, MosChip delivers world-class semiconductor design solutions. In a landscape where precision and efficiency are paramount, MosChip’s commitment to excellence is the compass guiding the future of power electronics.